The Alignment Research Center (ARC) has recently been studying the problem of Eliciting Latent Knowledge from AI agents. ARC has been holding contests to solicit proposals for solving this problem. What follows is my proposal to the February 15, 2022 version of the contest. [Update: this proposal received an honorable mention, and I’m interested in exploring this further.]
Basic training strategy and why it might work
The overall strategy is to avoid training a “human simulator” reporter by regularizing its internal state to have mind-blindness. One could imagine training a “Human Simulator” that takes as input the “what’s going on” state, plus a question about what a human believes about the world, and is trained to maximize its accuracy at predicting the human’s answer explanation. Meanwhile, the Reporter is trained with dual objectives: in addition to (as before) answering questions about the world correctly, it should be penalized for containing internal state that is useful to the Human Simulator. To do this, an auxiliary “Human Simulator Detector” model is trained to predict the Human Simulator’s activations from the Reporter’s activations. The Reporter is trained adversarially to minimize the Human Simulator Detector’s accuracy. The reporter would be trained not merely to be bad at predicting the explanation — an evil reporter could pretend to be bad at predicting the explanation — but to not contain state that is predictive of “human simulator state”. This is something that the various defeated strategies are missing. A devious reporter can easily hide its full knowledge from its predicted answer, and may even choose weights to look like it does not contain a human simulator. But we argue that when operating on actual input data, a devious reporter will find it more challenging to run a simulation while pretending not to.
This strategy is depicted below:
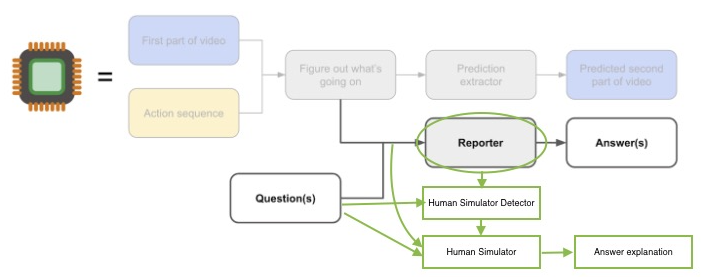
More precise detail on strategy
The Reporter’s overall loss function to minimize takes the form:
reporter_loss
= reporter_prediction_loss
– alpha
* human_simulator_detector_loss
.
How to find a strategy for choosing the hyperparameter alpha is an open question. But, if the Reporter can do just as well at reporting with and without containing a human simulator, then any positive alpha would be sufficient to choose not to contain a human simulator.
The Human Simulator assumes a form of data augmentation that allows us to train a model that knows how humans would interpret their observations of the world. In one embodiment depicted above, the human labeler will provide not only an answer, but an answer explanation. The Human Simulator would have the same inputs as the Reporter, but would predict this answer explanation rather than the answer. (Other possibilities can be envisioned. For example, the Human Simulator could be trained to predict both the answer and the answer explanation.)
The Human Simulator Detector takes in the Reporter’s internal state (activations) as input, and tries to predict the Human Simulator’s internal state (activations) as input (by training with MSE loss, for example). The Human Simulator Detector actually tries to minimize the
human_simulator_detector_loss
= simulator_state_mse_loss
+ gamma
* ||human_simulator_detector.weights
||^2,
where the latter term can be replaced with some standard approach to minimizing its model complexity. If the Reporter contains a human simulator within itself identical to the Human Simulator, then the Human Simulator Detector will be able to predict the Human Simulator state by simply selecting such states (ie it will be the identity function for the portion of the Reporter that is its human simulator). Note that if the Reporter contains its human simulator activations in a permuted order from the Human Simulator, and if the Human Simulator Detector is a single-layer feedforward network, then it could simply learn a permutation matrix rather than the identity matrix.
On the other hand, if the Reporter does not contain a human simulator within itself, the Human Simulator Detector will either have high model complexity (simulating a human presumably requires a complex model — especially because it is not given “what’s going on” as input, but instead on the Reporter’s internal state, which contains less information according to the Data Processing Inequality), or it will have high simulator_state_mse_loss.
Thus, the Reporter can be trained in an adversarial fashion, minimizing its own reporter_loss
, while maximizing the human_simulator_detector_loss
.
Counterexample
While I do not have a specific counterexample scenario that defeats this strategy, a “recipe” for a successful counterexample can be abstractly described. The scenario must be on in which it is easier for the Reporter to both learn its own simulator while also learning to disguise its simulator from the Human Simulator Detector, rather than learn to be an accurate reporter without learning a simulator. It would seem that standard regularization techniques applied to the Reporter would prefer the latter. So a counterexample would be one in which an accurate reporting would be difficult or impossible without human simulation.
Besides the possibility of counterexamples, this strategy could fail if one is unable to successfully train a Human Simulator Detector in the first place. To succeed we need to solve two problems that appear to be solvable yet practically challenging: training the Human Simulator, and training the Human Simulator Detector using the Human Simulator.
With regards to training the Human Simulator, the key challenge is obtaining labeled data that forces a model to not only reason about the video input, but also reason about how humans reason about the video input.
With regards to training the Human Simulator Detector, the model will have to map from a large input space to a large output space. However, because n_activations ~ sqrt(n_weights)
in a feedforward neural network, the Human Simulator Detector would probably require roughly the same number of weights as the two other networks. We assume that the Human Simulator Detector can be trained to be permutation invariant with respect to Reporter activations. This is not as hard as it looks: as noted in the previous section, so long as the permutation of activations is the same across samples, then undoing this is a sparse linear transformation. If the permutation of activations varies among samples, then this would be harder.
This was originally published here: https://calvinmccarter.wordpress.com/2022/02/19/mind-blindness-strategy-for-eliciting-latent-knowledge/
#ML #futurism